Estimating Future Customer Demand using Demand Forecasting
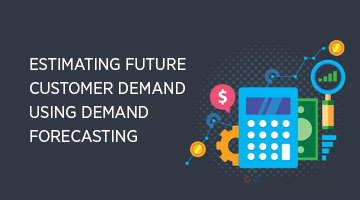
Introduction
Demand forecasting is critical to businesses across almost all industries. It is a process in which historical sales data is used to predict future customer demand. It provides an estimate of the amount of goods and services that customers will purchase in the foreseeable future. Critical business assumptions like turnover, profit margins, cash flow, capital expenditure, risk assessment, capacity planning, etc. are dependent on demand forecasting.
How is demand forecasting done?
- Defining the objective:
This task involves the following:
- Deciding the time period for which forecasting should be done (short-term or long-term).
- Deciding whether to forecast overall market demand for a product or for a particular organisation’s products only.
- Deciding whether to forecast demand for a market or for a particular market segment.
- Deciding whether to forecast market share for an organisation.
- Determining the time period:
The time period for forecasting is decided. Demand can be forecasted either for a short or a long period. This is decided on the basis of the objective set by an organisation.
- Selecting a method:
The method of forecasting differs from one organisation to another based on purpose, time-frame, data requirements and data availability.
- Data Collection:
Data collection can be either of the primary type or the secondary type. Primary data refers to data that is collected through observation, interviews and questionnaires. Secondary data refers to data that was collected in the past.
- Estimating the results:
It involves making an estimate of the forecasted demand. The results should be easily interpretable and presented in a usable form. The results should be easy to understand.
Types of demand forecasting
Short-term forecasting
It covers a short period of time depending on the nature of the business. It generally covers 6 months or less than a year. It is used for making tactical decisions.
Long-term forecasting
It is usually done for 2 to 5 years. It is used for making major strategic decisions.
Forecasting tools
Demand forecasting can be done using time-series analysis, which aims to detect patterns in the data and extend those patterns as predictions. The ARIMA (Auto-Regressive Integrated Moving Average) model is used for understanding the patterns and creating predictions; tree algorithms like CART (Classification And Regression Trees) also work well with such data. Different parameters can be used to detect linear, quadratic and constant trends.
Once adequate predictive models are found, they can be combined together to create a forecast, since a demand forecast model is usually an ensemble of many predictive models. A combination of models usually results in better predictive accuracy. Even if one model gets off track, the whole ensemble counteracts to nullify the effects. The model should be updated as and when new data is accumulated.
Conclusion
When demand forecasting is critical to a business and operational decisions are based on it, it is essential to have a robust and accurate forecasting model. Traditionally, demand forecasting has been done using time-series algorithms; time-series algorithms work well when the forecast horizon is short-term and the number of variables influencing demand is less. However, if the forecasting problem at hand does not conform to the above conditions, feature-based algorithms, which rely on abundant data, are preferable.