Data Science Use-Cases for Large Industries
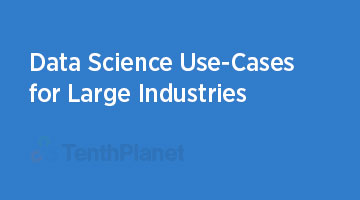
Data Science has a wide variety of applications. It is used in several fields ranging from health, education to transportation and manufacturing. Various industries are using Data Science to boost their production, make smarter decisions and develop innovative products that are tailored for customer needs. Let’s check how these industries are using Data Science.
Use Case
Industries Applicable
Up Selling and Cross Selling
Banking, Insurance, Retail, Telecommunications
Sentiment Analysis
Life Sciences, Education, Insurance, Retail, Telecommunications
Risk Modelling
Automotive, Banking, Manufacturing, Logistics & Transportation, Oil & Gas, Utilities
Quality Assurance
Automotive, Life Sciences, Manufacturing, Logistics & Transportation, Oil & Gas, Utilities
Propensity Modelling
Banking, Insurance, Retail
Predictive Modelling/Maintenance
Automotive, Manufacturing, Logistics & Transportation, Oil & Gas, Utilities
Customer Segmentation
Automotive, Banking, Life Sciences, Insurance, Retail, Telecommunications, Utilities
Next Best Action (Recommendation Systems)
Banking, Education, Insurance, Telecommunications
Customer Lifetime Value
Banking, Insurance, Retail, Telecommunications, Utilities
Churn Prevention
Automotive, Banking, Insurance, Retail, Telecommunications
Up Selling and Cross Selling
Predictive analytics can provide suggestions on which products might be combined to appeal to which market segments, to increase both your value to your customers, and the revenue derived from your customers.
Sentiment Analysis
Combining web search and crawling tools with customer feedback and posts, you can create analytics that give you a picture of your organisation’s reputation within your key markets and demographics, and provide you with proactive recommendations as to the best ways to enhance that reputation.
Risk Modelling
Predictive analytics can glean potential areas of risk from the massive number of data points collected by most organizations, and sorting through them to identify potential areas of risk, and trends in the data that suggest the development of situations that can affect the business and bottom line. By combining these analytics with a cogent risk management approach, companies can capture and quantify risk issues, evaluate them, and decide on a course of action to mitigate those risk factors deemed most critical.
Quality Assurance
Quality control is key to not just the customer experience, but also to your bottom line and operational expenses as well. Over time, inefficient quality control will affect your customer satisfaction, buying behaviors, and ultimately impact revenues and market share. Good predictive analytics, however, can provide insight into potential quality issues and trends before they become truly critical issues.
Propensity Modelling
Propensity models are often used to identify those most likely to respond to an offer, or to focus retention activity on those most likely to churn. The model may be applied to your database to score all your customers or prospects. You can then select only those who are most likely to exhibit the predicted behavior, for example response, and focus your mailing activity appropriately.
Predictive Modelling/Maintenance
By analyzing metrics and data related to the life-cycle maintenance of technical equipment, companies can predict both timelines for probable maintenance events and upcoming capital expenditure requirements, allowing them to streamline their maintenance costs and avoid critical downtime.
Customer Segmentation
Customer segmentation involves grouping customers into specific marketing groups, perhaps narrowing them down by gender, interests, buying habits or demographic. The process requires a thought-out strategy, understanding how to manage and group your customers and which data you will use to do this. By differentiating their customer base, businesses can better target individuals and maximize sales, link-sell appropriately and provide more tailored shopping experiences.
Next Best Action (Recommendation Systems)
Defining your primary market segments and customers is a critical use case for predictive analytics. But that only provides an incomplete picture of what your marketing approach should be. Analytics can also provide insight on the best way to approach individual customers within those segments, by analyzing everything from buying patterns to consumer behavior to social media interactions, giving you insight into the best times and channels to connect to those customers.
Customer Lifetime Value
One of the more difficult things to do in marketing is to identify those customers that are going to spend the most money, in the most consistent way and over the longest period of time. This kind of insight allows companies to optimize their marketing to increase their share of that segment of the business, and gain those customers that will have the greatest lifetime value to your company.
Churn Prevention
Customer churn occurs when customers or subscribers stop doing business with a company or service. Also known as customer attrition, customer churn is a critical metric because it is much less expensive to retain existing customers than it is to acquire new customers – earning business from new customers means working leads all the way through the sales funnel, utilising your marketing and sales resources throughout the process. Customer retention, on the other hand, is generally more cost-effective as you’ve already earned the trust and loyalty of existing customers.